核心技术
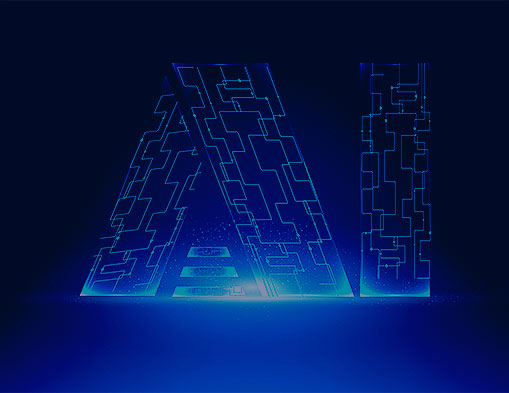
Deep Semantic Understanding
深度语义理解
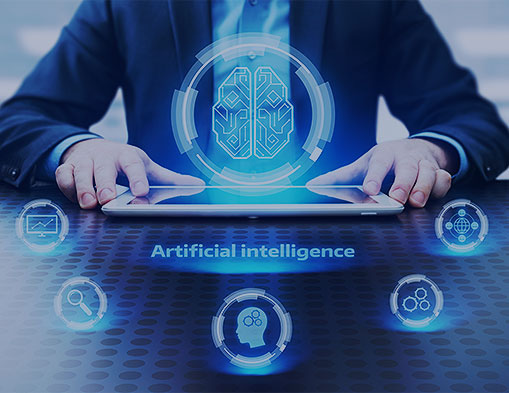
Social Computing
社会计算
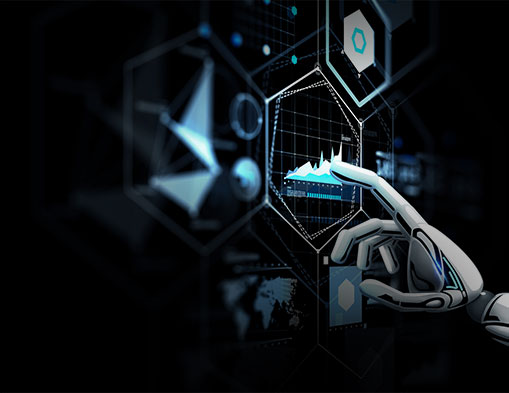
AI Engineering
人工智能工程
学术前沿
专利技术
校企合作
-
2023-07-14清华大学-中科闻歌清华x-lab“SDG开放创新马拉松挑战赛”
-
2021-12-12北京大学-中科闻歌教学科研虚拟仿真数据新闻实验室
-
2021-12-12中国科学院大学-中科闻歌“创新创业与企业实务训练营”走进闻歌
-
2021-12-12天津大学-中科闻歌计算智能联合实验室
-
2023-07-14北京师范大学-中科闻歌战略合作协议
-
2023-06-28西安交通大学-中科闻歌共建大数据与新闻传播实训实践基地
-
2023-07-14北京邮电大学-中科闻歌与北京邮电大学人工智能学院共同创建创新实践基地
-
2023-06-28西北大学外国语学院-中科闻歌专业学位研究生示范性实践基地合作单位
-
2023-07-14四川外国语大学-中科闻歌四川外国语大学人才培养计划